Many successful businesses today, whether they are online-based, brick and mortar or a combination of the two, utilize some sort of business big data management process to support important business functions such as storing customer information, tracking marketing and sales activity, and managing overall finances.
But beyond that, many businesses don’t do anything with all of the data that they collect and store. With predictive analytics, however, a company can meaningfully leverage that business data to diagnose and solve business problems.
Since NGDATA specializes in helping companies harness the power of data analysis to improve business success, we wanted to learn more about specific applications for predictive analytics. We specifically wanted to discover tips from predictive analytics experts on what specific ways predictive analytics tools and software solutions can effectively solve common business issues.
To do this, we asked 26 predictive analytics experts to answer this question:
“What’s the #1 business problem companies can solve with the help of predictive analytics?”
We’ve collected and compiled their expert advice into this comprehensive guide on how to use predictive analytics tools to benefit your business. See what our experts said below:
Meet Our Panel of Data Analytics Experts:
Stephen Timms
Dmitri Williams
Maria Casu
Will Hayes
Dean Malmgren
Pratibha Vuppuluri
David Cohen
Chuck Russell
Jeff Catlin
Nathan Gnanasambandam
Tesla Martinez
Charles “Drew” Settles
David Scarola
Jenna Puckett
Andrew Thomas
Jared Siegel
April Wilson
Ted Zollinger
Sandip Devarkonda
Ryan Naudé
Ian Aronovich
Elissa Fink
Majid Shaalan
Sean Higgins
Allyson Kuper
Michael Weiss
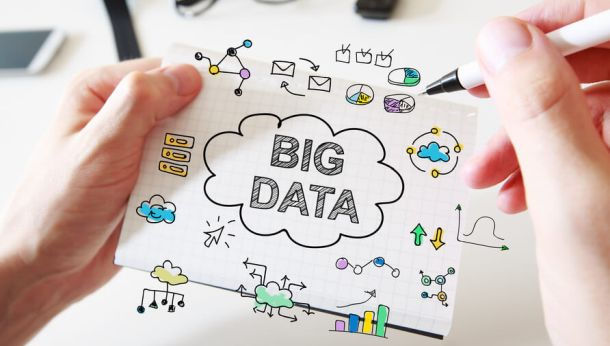
Stephen Timms
Stephen Timms is President of ClickSoftware, and has been with ClickSoftware for 9 years, rising to President of the Americas region in 2014. In his time at ClickSoftware he has led several teams through significant growth of customers, employees and revenue. He started his career teaching Networking and DataComms to post-grad students, and combines deep technical expertise to his vast sales skills and strong leadership style. He graduated Harvard Business School Executive Education – General Manager Program, in 2013.
Predictive capabilities let business take service to the next level and deliver a superior customer experience, pure and simple. Here’s how:
Planners can predict what next week’s (or next months’) schedule will look like, and get ahead of any potential gaps in coverage or capacity.
Predictive-powered appointment booking allows call center reps to provide customers with options for appointments that have a higher probability of success.
Schedulers and dispatchers can better focus on high risk tasks, consider predicted fluctuation in traffic during the day, and predict task duration based on measured individual efficiency.
Field resources can detect a certain physical contexts, analyze situations, and prompt a user to notify a customer when he is 15 minutes away from arriving to the service appointment.
For example, when an HVAC technician begins his week, predictive can compare the jobs for that day, and the next few days, to the parts available on his truck. If he needs three compressors and only has one, the app alerts him to the missing parts. The app will also know that a specific job type requires a specific part, and will prompt the tech to bring that part into the building. If a mobile worker has the right tools in hand every time when leaving the truck—that 10 minutes saved on each job is exponential, and easily equates to an extra job per day.
What we’re talking about is a new generation of empowered field workers. Where data-driven decision making had been reserved to the back office for back office users only, predictive brings it to the user on the front end. When organizations harness predictive analytics as a way that’s both cost effective for the company, and not overly invasive for either the customer or employee, the customer rises to the top like never before.
Dmitri Williams
Dmitri Williams (PhD, University of Michigan) is the CEO, Sensei, and Co-Founder of Ninja Metrics, Inc. Dmitri is a 15-year veteran of games and community research, and a world-recognized leader in the science of online metrics and analysis. The author of more than 40 peer-reviewed articles on gamer psychology and large-scale data analysis, Dmitri‘s work has been featured on CNN, Fox, the Economist, the New York Times, and most major news outlets. He has testified as an expert on video games and gamers before the U.S. Senate, and is a regular speaker at industry and academic conferences.
The #1 business problem companies can solve with the help of predictive analytics tools is…
Predictive analytics can give a developer or marketer unprecedented data to help them understand more about users, including Social Value.
We all know that people influence each other. If your family or friends do something, your chances of doing it go up–whether it’s playing a game, eating tofu, or smoking. This kind of influence can not only be predicted, it can be measured. And if that extra playing, or spending, or eating leads to dollars, that’s a value you can capture and impact. We call this “social value™” and it is responsible for between 20% and 50% of all spending.
If you have social value metrics, you have three ways to take advantage of them. First, you try to acquire more players like them. You can use our funnel analytics to know how valuable players from it really were. Second, you can leverage your influencers with promotions and see which cause more spending among their friends. Third, you know who to focus your retention efforts on. Maybe that player doesn’t seem important because they don’t spend a lot, but if they go, you’ll lose $300 from their friends. Social value analytics will go a step further and tell you which mechanics or experiences are leading to higher or lower values.
Maria Casu
Maria Casu is the Head of Marketing at Portal, a business and technology consultancy.
Depending on the business model the company has adopted, the chief (and most valuable) use of predictive analytics is to…
Identify individuals who have the highest propensity to buy, or alternatively, to identify those existing customers who have the highest propensity to leave.
This is largely being driven by today’s “empowered customers” who expect to have a consistent experience across the brands they are doing business with. Instant visibility of customer sentiment via social media coupled with unlimited access to alternative products and suppliers has resulted in a power flip between buyer and seller. Raised expectations about product, price, delivery and service have drastically shrunk the window of time for merchants and service providers to respond to customer interactions and convert to orders.
Traditionally, getting close to customers was seen as a priority for sales and marketing alone, but senior executives are starting to understand that the customer must be front and centre of every employee in the organisation. Understanding the customer is now everybody’s job – from R&D and manufacturing right through to point-of-sale and all post-sale service teams, including accounts, renewals, field service, and so on.
As a result, predictive analytics is being used to uncover hidden insights in customer data to create much more personalised experiences, whilst also enabling the business to operate more effectively by:
Identifying hidden patterns and predictive models to target campaigns, promotions and offers;
Using churn models to predict which customers are at risk of leaving and identify any clues about why;
Measuring the likely lifetime value of customers to improve sales forecasting and profitability; and
Ascertaining customer sentiment to spot emerging trends.
In this way, predictive customer intelligence can yield impressive results for businesses which are committed to growing, retaining and satisfying customers by improving their interactions and business strategy across multiple channels.
Will Hayes
Will Hayes is the CEO of enterprise search company Lucidworks. In his leadership role, Will Hayes brings search, discovery and data analytics solutions to companies all over the world. Lucidworks is solving the problems presented by ‘Big Data’ with tools for the enterprise built on open source Solr technology. Hayes has 15 years of product, marketing and business development experience, most recently as the head of technical business development for Splunk, an industry leader in enterprise data analytics.
The #1 business problem companies can solve with the help of predictive analytics tools is…
Making sense of large amounts of unused business data.
With the diminishing cost of cloud storage, companies are amassing more data than ever before. While simple data collection is fine, the reality is that most companies only use about 1 percent of their stored information to translate into actual business decisions.
Why? It’s because they can’t find their data. The ability to search and retrieve information is the most important action for scaling your business and realizing the promise of big data. And that search technology needs to be fast, contextual and programmed to read natural language so it’s usable for employees at every level of an organization.
Dean Malmgren
Dean Malmgren is a Co-Founder and Data Scientist at Datascope Analytics, a data-driven consulting and design firm in Chicago, where he has helped clients like P&G, Daegis, and Thomson Reuters use data to solve the right problem. Dean received a BS from the University of Michigan and a PhD from Northwestern University, has spoken at conferences like Strata, and published peer-reviewed research in journals like Nature, Science, and PNAS, that have been featured in places like TIME, Wired, and US News & World Report.
The #1 business problem companies can solve with the help of predictive analytics is…
Inaccurate or misleading revenue forecasts and models.
Businesses frequently forecast revenues with extraordinarily rough models. These models are often the boilerplate year-over-year growth from a QuickBooks forecast or sometimes even built in Excel. Most importantly, these models almost always spit out a single point estimate for each month going forward. This is a huge mistake because, as every business owner knows, revenues can fluctuate wildly and we have great uncertainty about next month, let alone the next quarter or year.
Data science offers a unique solution to this challenge. By properly accounting for the particular mechanisms by which companies generate revenue and the uncertainty in sales, businesses can gain much more nuanced revenue forecasts that not only give you the best estimate revenue for the next month, quarter or year, but can also give transparency into the variability of the different revenue outcomes—the good and the bad.
Pratibha Vuppuluri
Pratibha Vuppuluri is the Founder & CEO at KeyInsite Inc., a data analytics company. She is former Investment Banker with over 10 years experience and has authored an academic publication on “The Impact of Negative Economic News Coverage on Consumer Confidence”. Pratibha hold a Masters degree from Columbia and has completed her undergraduate from Cornell University.
The #1 business advantage of predictive analytics is…
Lowering marketing promotion campaign costs and increasing the ROI from these campaigns.
The need for increased online brand awareness through an optimized digital marketing spend is a crucial factor to most companies’ success. To deliver on promotion $, all digital promotional campaigns should have pre-event targets and predictions to project and measure against. The 3 vital components to estimate promotion dollars are a) the projected exposure, b) the average value of a response, and c) the predicted response rate:
*Promotion $ = Exposure x Average Redemption Value x Redemption Rate*
Exposure
Exposure is a factor of how many people, a campaign would reach via the channels (mobile, online, social…) on which the message is delivered. Based on the objective of the marketing effort, it is vital to predict the type of exposure a campaign would receive in channels selected.
Average Redemption Value (ARV)
Average Redemption Value is the net margin a campaign would deliver when a customer acts on it. The ARV is quite often a factor of the product category and the average ticket size of the customer transacting in the promotion. Patterns such as while a digital customer’s average propensity to spend is higher, he is more likely to ‘price shop’ and hence the promotions should be meaningful and value worthy is of essence.
Redemption Rate
Perhaps the most challenging of them all is to predict the redemption rates. Partly because channel open rates are highly volatile, susceptible to erratic consumer online behavior and subject to macro-factors. Using ‘like-event’ modeling with statistical confidence interval approach provides a directional approach to estimate redemption rates of segments of the target audience.
To deliver on the promise of promotional ROI, all these factors can be predicted using historical data to give marketers an advantage in lowering promotion campaign costs and increasing the return from these campaigns.
David Cohen
David Cohen is the Director of Information at Shutterstock, a leading provider of digital imagery licensing, operating in more than 150 countries and 20 languages.
In general, when looking for problems to apply predictive analytics, I encourage my analysts to…
Focus on micro-decisions.
Too often, analysts get caught up in trying to solve relatively large problems with predictive analytics. Focusing on the small decisions that are made thousands of times tends to yield better results, in my experience. These include things like what checkbox to pre-check, what item they are most likely to buy, or what parts of the site a user is most likely use. While improving these can yield relatively small percentage gains, they happen so frequently that the total improvement can be high.
Comments